Climate Impacts on Drinking Water: Data and Methods
Introduction
This page describes the data and methods used to develop 1) projections of future surface water supply from selected source water basins, and 2) projections of municipal (drinking) water demand for selected water districts, on the Oregon Mid-Coast.
For assessing future water supply, changes in monthly streamflow relative were projected to 2070 using computer models of basin hydrology. The hydrological models were fed with simulated future daily temperature and precipitation consistent with a climate that is changing due to increasing greenhouse gases (GHGs). Changes in both the long-term mean monthly flow and the 10th percentile of flow (the low flow that would be reached 1 in 10 years, on average) were projected.
For assessing future water demand, demand was assumed to be affected by the combination of population growth and climate change. Demand was first projected to grow at the same percentage rate as the projected population to the year 2070. A “climate effect” was then added to the population-adjusted demand. The climate effect was determined in two steps. First, we estimated the sensitivity of monthly demand to weather using historical records of water demand and weather. The “sensitivities” effectively tell us how much demand changes with a change in, for example, temperature. Second, the sensitivities were applied to simulated future daily temperature and precipitation consistent with a climate that is changing due to increasing GHGs to produce climate-driven changes in demand into the future.
The remainder of this page describes the methods in detail.
Projected changes in water supply
Siletz River Basin only
For the Siletz River basin, we used computer simulations of streamflow developed by Burke and Ficklin (2017) who used a hydrologic model called the Soil and Water Assessment Tool (SWAT; Arnold et al. 1998). The SWAT model represents the land with irregular polygons that represent the many sub-basins that compose the larger Siletz basin. The sub-basins range in size, with the smallest being < 1 km2 in area. The SWAT model includes the Siletz basin above the USGS station on the Siletz River at Siletz, OR (Station ID 14305500), The simulations span the years 1953 – 2099 and were generated using meteorological inputs from 20 global climate models (GCMs) (see Table 1). Most of the GCMs were run twice, with one run assuming a future with a moderate increase in GHG concentrations and the second run with a large increase in GHG concentrations, by the end of the 21st century. The moderate and high GHG scenarios are known as Representative Concentrations Pathway 4.5 (RCP 4.5) and 8.5 (RCP 8.5), respectively (Van Vuuren et al. 2011).
The meteorological inputs to SWAT, specifically daily precipitation and daily minimum and maximum temperature, from the GCMs were downscaled to 1/8-degree resolution. The downscaling was done using a statistical method known as Bias Corrected Constructed Analogues (BCCA; Mauer et al. 2010; U.S. Bureau of Reclamation 2013). The downscaled data were accessed at https://gdo-dcp.ucllnl.org/downscaled_cmip_projections.
The SWAT model was calibrated against measured streamflow at the Siletz River station with generally good results, especially for higher streamflow values (Burke and Ficklin, 2017), However, we observed systematic biases in low flows. For example, the simulated streamflow tended to decline too quickly in the summer, and reached levels that were too low by August. Also, streamflow tended to rise too quickly in September and October with the onset of autumn rains.
Because summer and early autumn low flow periods are so critical to water supply, we applied a bias-correction procedure to the simulated streamflow. Using a parametric quantile-mapping method, we were able to reduce errors in low flows. A detailed description of the bias-correction method is in the Appendix.

We averaged daily basin discharge (surface plus groundwater) by month. Then, we calculated two statistics for each calendar month: 1) the long-term mean and 2) the 10th percentile of monthly discharge. Both statistics were calculated over 30-year windows, with each window offset by ten years. We then averaged each statistic over all ten GCMs per RCP. Averaging over the GCMs has two benefits: 1) it smooths out some of atmospheric variability that is not driven by the increasing GHGs but which can otherwise cause the effect of climate change to appear larger or smaller than it actually is, and 2) it can help offset errors in the individual GCM simulations.
The period 1991-2020 was used as a reference period and changes in each statistic with respect to the reference period were calculated for other 30-year periods.
Other Coastal Basins
For coastal basins other than the Siletz River basin, we used computer simulations of basin discharge developed as part of the Columbia River Climate Change (CRCC) project funded by the Bonneville Power Administration, USACE, and Bureau of Reclamation (RMJOC, 2018; Chegwidden et al. 2019). The CRCC simulations cover much of northwestern United States, span the years 1950 – 2099, and were generated using 20 distinct modeling configurations and therefore consist of 20 separate 150-year long simulations of basin discharge.
The 20 simulations are the results of running one hydrological model but with meteorological inputs derived from ten GCMs that are listed in Table 1. Each GCM was run twice, once under the RCP4.5 scenario a second time under the RCP8.5 scenario.
The Variable Infiltration Capacity model (VIC version 4.2.glacier.01; Liang et al., 1994) was used to model the hydrology of the basins. The VIC model represents the land with a grid composed of cells that are 1/16-degree latitude by 1/16-degree latitude in size (about 6 km). The meteorological data, specifically daily precipitation and daily minimum and maximum temperature, from the GCMs were downscaled to 1/16-degree resolution to be consistent with the hydrological model. The downscaling was done using a statistical method known as Multivariate Adaptive Constructed Analogs (MACA; Abatzoglou and Brown, 2012). Specifically, the version of MACA data used are called “MACAv2-Livneh” and were accessed at https://www.climatologylab.org/maca.html.
The 1/16-degree gridded hydrological model output was mapped onto polygons representing the basins. Basin discharge was calculated as an area-weighted sum of the grid cell discharge, where the weights were proportional to the area of the basin that overlapped each grid cell. Because of the relatively coarse resolution of the VIC model, not all basins that serve as water sources for the major Mid-Coast water suppliers could be represented.
Finally, we averaged daily basin discharge (surface plus groundwater) by month. Then, we calculated two statistics for each calendar month: 1) the long-term mean and 2) the 10th percentile of monthly discharge. Both statistics were calculated over 30-year windows, with each window offset by ten years. We then averaged each statistic over all ten GCMs per RCP. The period 1991-2020 was used as a reference period and changes in each statistic with respect to the reference period were calculated for other 30-year periods.
Due to the scarcity of streamflow measurements in most basins that serve as drinking water sources in the Mid-Coast, evaluating the accuracy of the VIC model simulations was not feasible. Therefore, care should be taken when using these simulations.
Projected Changes in Water Demand
Data
We acquired historical daily or monthly municipal water production for five water districts in the Oregon Mid-Coast (Table 2). Daily production was aggregated to monthly production. For a sixth water district (City of Waldport), we acquired monthly metered water consumption, separated by indoor and outdoor consumption. Although water production data were available for Waldport, the data record was very short (3.75 years), whereas the consumption data spanned five years. The length of record varied by district and ranged from five to 33 years.
Production is defined as the total water delivered from a water treatment plant to meet the demand of users, along with transmission losses and unmetered water use. Transmission losses plus unmetered usage vary by district and time, but estimates range from 6 to 22% of total water produced by the treatment plants.

We acquired historical meteorological data from the PRISM Climate Group (Daly et al. 2008, 2021; https://prism.oregonstate.edu/): daily precipitation, daily maximum temperature (Tmax), and daily minimum temperature (Tmin). The PRISM data are provided on a 1/24°(~4-km)-resolution grid so we extracted data for the grid cells closest to the center of each water district. We also extracted data for the cells closest to the Portland International Airport (PDX), Eugene Airport (EUG), and Salem Municipal Airport (SLE). These three locations represent the main population centers (Portland Metropolitan Region, Eugene-Springfield, and Salem) in the Willamette Valley of Oregon, and we assumed would be the source of most tourist visits to the Mid-Coast.
We also acquired simulations of daily meteorological variables under two scenarios of future greenhouse gas concentrations: Representative Concentration Pathway (RCP) 4.5 and 8.5 (Van Vuuren et al., 2011). RCP 4.5 and RCP 8.5 are often considered moderate and high-end emissions scenarios, respectively. The GHG concentrations assumed by RCP 8.5 towards the end of the twenty-first century are currently considered unlikely (Hausfather and Peters 2020) but still plausible over the next few decades (Schwalm et al 2020).
The simulated daily precipitation, Tmin, and Tmax were generated with 20 GCMs listed in Table 3 that were used in the Coupled-Model Intercomparison Project Phase 5 (CMIP5; Taylor et al., 2012). The daily GCM output for the years 1950 – 2099 was statistically downscaled using the Multivariate Adaptive Constructed Analogs Method (MACA; Abatzoglou and Brown, 2012). Like the PRISM data, the MACA data are gridded with a resolution of 1/24 degree. We used the data from MACA and PRISM at the same geographic locations. The MACA data were acquired from the Climatology Lab at the University of California, Merced at http://www.climatologylab.org/maca.html.
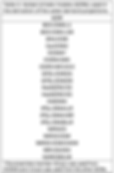
Historical population records and future population forecasts were obtained from the Portland State University Population Research Center. Our analysis uses populations forecast prepared in 2017 and downloaded from https://www.pdx.edu/population-research/. New forecasts for Lincoln County have recently been made available so we may update our analysis.
Methods
Water demand is defined as the total amount of water extracted from the water supply to meet the demand of the municipal water system. We used water treatment plant water production as an estimate of total demand, except for Waldport where we used consumption data, knowing that consumption is less than production. Where we refer to “demand” below, it has been estimated with either production or consumption.
We estimated the sensitivity of monthly water demand to weather using historical water demand and weather variables. A sensitivity is defined as a percentage change in demand for a given change in some other variable, such as temperature. We applied these sensitivities to projections of future changes in weather variables to estimate changes in water demand due to climate change.
Sensitivities of water demand to weather variables were estimated using ordinary least squares regression. Monthly water demand was statistically modeled as a function of weather and other factors that allowed for a non-linear response of water demand to weather (Dundas and von Haefen, 2020). Daily Tmin and Tmax were transformed by summing the number of days in a month that the variable fell within a bin spanning a range of values. For both Tmin and Tmax, we constructed bins in 10°F intervals and each bin contained the count of the number of days per month when the temperature fell within that specific bin. For precipitation, we summed the number of days with no measurable precipitation (daily total < 0.01 inches) within each month and calculated the total precipitation over each month. Intuitively, the model coefficients on the bins allowed us to identify how an additional hot, cold, dry, or wet day affects water demand. The statistical model also included the effects of holidays and weekends measured as the count of holiday days or weekend days in each month. The quarter of the year (i.e., January – March, April – June, July – September, and October – December) was included as a factor in the model, as was the year so that time-varying demand resulting from changes in historical water use efficiency or population could be accounted for implicitly.
Statistical models were built for each water district. In the case of Toledo, we subtracted Seal Rock Water District’s water demand from Toledo’s water demand because until 2023, Toledo production included sale of water to Seal Rock Water District. Until very recently, Seal Rock Water District obtained all of its water from Toledo but now gets its water from Beaver Creek. Demand data were also pooled over all districts except Toledo to build a model that represented the coastal communities on the Mid-Coast in general. Toledo was excluded because, unlike the other locations, it is not considered a tourist destination.
An initial analysis revealed little sensitivity of demand to local weather. This is not surprising, given the relatively temperate climate on the Oregon Coast moderated by the Pacific Ocean. We then tested a hypothesis that coastal towns and water providers may be sensitive to an influx of people from tourism. In other words, mild coastal cities likely see a large increase in visitors (and demand for water) when temperatures are hot in the nearby Willamette Valley. We replaced local weather variables with Tmin, Tmax, and precipitation representative of the Willamette Valley by averaging weather variables for PDX, SLE, and EUG. We found that water demand was sensitive to Willamette Valley Tmax in all districts. Figure 1 illustrates the sensitivity Newport water demand to Willamette Valley Tmax. This figure shows, for example, that for every day in the month that the temperature falls between 90 and 100°F, the monthly water demand increases by 2.5% above a baseline demand.
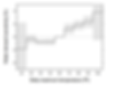
Figure 1. Sensitivity of Newport, OR, monthly water demand to the number of days in a month that the daily maximum temperature (Tmax) falls with specific ranges. Shaded bars show 95% confidence limits.
We projected future water demand as the combined effect of population growth and climate change. We calculated demand representing “current conditions” (~2021) by averaging demand over the maximum of either the last five years of record or the length of the available record. The ”2021” demand was calculated separately for each calendar month. To estimate this 2021 demand. we used water production for all districts, including the 3.75 years of production data for Waldport. To adjust future demand for population growth, with multiplied the 2021 demand by the forecasted population in each year from 2021 to 2070 and divided by the population estimated for 2021. We assumed no changes in water use efficiency over time, although increases in water use efficiency are likely to occur.
To account for climate change, we first applied the sensitivities to the simulated weather from the MACA dataset to calculate the response of demand to future climate conditions. The results were monthly time series of “climate-adjusted-only” water demand from 2001 – 2070 corresponding to each of 20 GCMs and two RCPs (i.e., 40 time series). Monthly demand anomalies were calculated as percentage differences from the 2001 - 2030 climatological average of demand.
We multiplied the population-adjusted mean demand for each month of each year by the water demand anomalies (divided by 100) described above. This provided 40 time series of water demand projections. To both help cancel potential errors among the GCMs and smooth out climate variability not driven by increasing greenhouse gas concentration, we averaged the water demand projections across the 20 GCMs for each RCP. We smoothed the GCM-averaged projections yet further with a cubic-spline smoothing algorithm.
Appendix: Bias-correction of daily streamflow
We compared statistics of observed and simulated daily streamflow at the USGS station on the Siletz River at Siletz, OR (Station ID 14305500). Although the SWAT model generally reproduced the shape of annual hydrograph, the simulations had some systematic biases (Figure A1). For example, the simulated median daily flow was too low in late summer and too high in autumn. Also, the model tended to generate daily low flows (e.g., 5th percentile) that were lower than observed in August and September, but daily low flows that were too high for late autumn and winter.

Figure A1. Observed (obs.) and simulated (model) streamflow statistics by day of year for the Siletz River at Siletz, OR for the years 1970–2019. The heavy dashed and solid lines show the median of the observed and simulated streamflow, respectively. The lighter dashed lines show the 5th and 95th percentiles of the observed streamflow. The shaded region spans the 5th to 95th percentile of the simulated streamflow.
We used a quantile-mapping (QM) bias-correction method (e.g., Ines and Hansen 2006; Piani et al, 2010, Gudmundsson et al 2012) to transform the simulated data to be more statistically consistent with the observations. The transformation of the simulated data is defined as
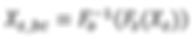
where Xs is the variable to be transformed (i.e., the simulated streamflow data), Fs is the cumulative distribution function (CDF) of Xs,
Fo-1 is inverse CDF (i.e., quantile function) of the observed variable Xo, and Xs_bc is the transformed (i.e., bias-corrected) value of Xs.
We assumed theoretical CDFs (Fo and Fs) for the observed and simulated daily streamflow. We first tried both the two-parameter Weibull and log-normal distributions but settled on a combination of the log-normal distribution and the three-parameter skew-log-normal distribution. The skew-log-normal distribution is identical to the log-normal distribution when the skew-log-normal shape parameter = 0.
We allowed the distribution parameters to vary by calendar day. The parameters were estimated separately for each calendar day (January 1 through December 31) using data within a 31-day window centered on the target calendar day for all years from 1954 through 2018 (after removing February 29). The last fifteen days of 1953 and first 15 days of 2019 were added the beginning and end, respectively, of the 1953 – 2018 time series. From the observed streamflow, each parameter set was therefore estimated using 31 days × 65 years = 2,015 values. From the simulations, we pooled all data using all the GCM inputs (19 GMCs) per RCP, so each parameter set was estimated using 31 days × 65 years × 19 GCMs = 38,285 values. By pooling all the GCMs, we effectively assumed each simulation from each GCM was equally likely and all came from the same underlying population.
The above procedure produced 365 parameter sets from the observations and another 365 parameter sets from the simulations for each RCP. To avoid sharp changes in parameter values from day to day, we used a cubic-spline smoother to smooth the time series of each parameter.
A large and relatively sharp change in the shape of the distribution occurred in the latter half of October, coinciding with the large increase in streamflow from about mid-October to mid-November. Parameter instability using the skew-log-normal distribution for this period of large changes in streamflow was an issue. To help alleviate the problem, we used bias-corrected streamflow values derived using the skew-log-normal distribution before October 21 and values using the log-normal distribution after October 27. Between October 21 and 27, we used an average of the values derived from each distribution.
The bias-correction was done using R Statistical Software (R Core Team 2021) with the “sn” package (Azzalini 2023) for applying the skew-log-normal distribution, the “MASS” package (Venables and Ripley 2002) for applying the log-normal and Weibull distribution, and “mgcv” package (Wood 2004) for the temporal smoothing of the parameters.
As shown in Figure A2, the bias-correction simulations more closely match the observations both in the median and extremes (5th and 95th percentiles) throughout the year. Satisfied with these results, we bias-corrected all the streamflow simulations out the year 2100.

Figure A2. Observed (obs.) and bias-corrected simulated (model) streamflow statistics by day of year for the Siletz River at Siletz, OR for the years 1970 – 2019. The heavy dashed and solid lines show the median of the observed and bias-corrected simulated streamflow, respectively. The lighter dashed lines show the 5th and 95th percentiles of the observed streamflow. The shaded region spans the 5th to 95th percentile of the bias-corrected simulated streamflow.
References
Abatzoglou, J. T., & Brown, T. J. (2012). A comparison of statistical downscaling methods suited for wildfire applications. International Journal of Climatology, 32(5), 772–780.
Arnold, J. G., Srinivasan, R., Muttiah, R. S., & Williams, J. R. (1998). Large area hydrologic modeling and assessment part I: model development 1. JAWRA Journal of the American Water Resources Association, 34(1), 73-89.
Azzalini, A. (2023). The R package 'sn': The Skew-Normal and Related Distributions such as the Skew-t and the SUN (version 2.1.1), http://azzalini.stat.unipd.it/SN/,https://cran.r-project.org/package=sn
Burke, W. D., & Ficklin, D. L. (2017). Future projections of streamflow magnitude and timing differ across coastal watersheds of the western United States. International Journal of Climatology, 37(13), 4493-4508.
Chegwidden, O. S., Nijssen, B., Rupp, D. E., Arnold, J. R., Clark, M. P., Hamman, J. J., ... & Pan, M. (2019). How do modeling decisions affect the spread among hydrologic climate change projections? Exploring a large ensemble of simulations across a diversity of hydroclimates. Earth's Future, 7(6), 623-637.
Daly, C., Halbleib, M., Smith, J. I., Gibson, W. P., Doggett, M. K., Taylor, G. H., ... & Pasteris, P. P. (2008). Physiographically sensitive mapping of climatological temperature and precipitation across the conterminous United States. International Journal of Climatology: a Journal of the Royal Meteorological Society, 28(15), 2031-2064.
Daly, C., Doggett, M. K., Smith, J. I., Olson, K. V., Halbleib, M. D., Dimcovic, Z., ... & Kaspar, E. M. (2021). Challenges in observation-based mapping of daily precipitation across the conterminous United States. Journal of Atmospheric and Oceanic Technology, 38(11), 1979-1992.
Dundas, S. J., & von Haefen, R. H. (2020). The effects of weather on recreational fishing demand and adaptation: Implications for a changing climate. Journal of the Association of Environmental and Resource Economists, 7(2), 209-242.
Gudmundsson, L., Bremnes, J. B., Haugen, J. E., & Engen-Skaugen, T. (2012). Downscaling RCM precipitation to the station scale using statistical transformations–a comparison of methods. Hydrology and Earth System Sciences, 16(9), 3383-3390.
Hausfather, Z., & Peters, G. P. (2020). Emissions – the ‘business as usual’ story is misleading. Nature, 577, 618–620.
Ines, A. V., & Hansen, J. W. (2006). Bias correction of daily GCM rainfall for crop simulation studies. Agricultural and Forest Meteorology, 138(1-4), 44-53.
Liang, X., Lettenmaier, D. P., Wood, E. F., & Burges, S. J. (1994). A simple hydrologically based model of land surface water and energy fluxes for general circulation models. Journal of Geophysical Research: Atmospheres, 99(D7), 14415-14428.
Maurer, E. P., Hidalgo, H. G., Das, T., Dettinger, M. D., & Cayan, D. R. (2010). The utility of daily large-scale climate data in the assessment of climate change impacts on daily streamflow in California. Hydrology and Earth System Sciences, 14(6), 1125-1138.
Piani, C., Haerter, J. O., & Coppola, E. (2010). Statistical bias correction for daily precipitation in regional climate models over Europe. Theoretical and Applied Climatology, 99, 187-192.
R Core Team (2021). R: A language and environment for statistical computing. R Foundation for Statistical Computing, Vienna, Austria, https://www.R-project.org/
River Management Joint Operating Committee (RMJOC) (2018). Climate and Hydrology Datasets for RMJOC Long-Term Planning Studies, Second Edition: Part 1 – Hydroclimate Projections and Analyses. Retrieved from https://usace.contentdm.oclc.org/digital/collection/p266001coll1/id/10562
Schwalm, C. R., Glendon, S., & Duffy, P. B. (2020). RCP8.5 tracks cumulative CO2 emissions. Proceedings of the National Academy of Sciences, 117(33), 19656-19657.
Taylor, K. E., Stouffer, R. J., & Meehl, G. A. (2012). An overview of CMIP5 and the experiment design. Bulletin of the American Meteorological Society, 93(4), 485–498.
U.S. Bureau of Reclamation (2013). Downscaled CMIP3 and CMIP5 Climate and Hydrology Projections: Release of Downscaled CMIP5 Climate Projections, Comparison with preceding Information, and Summary of User Needs, prepared by the U.S. Department of the Interior, Bureau of Reclamation, Technical Services Center, Denver, Colorado. 47pp.
Van Vuuren, D. P., Edmonds, J., Kainuma, M., Riahi, K., Thomson, A., Hibbard, K., & Masui, T. (2011). The representative concentration pathways: an overview. Climatic Change, 109(1–2), 5.
Venables WN, Ripley BD (2002). Modern Applied Statistics with S, Fourth edition. Springer, New York. ISBN 0-387-95457-0, https://www.stats.ox.ac.uk/pub/MASS4/
Wood, S.N. (2004) Stable and efficient multiple smoothing parameter estimation for generalized additive models. Journal of the American Statistical Association. 99:673-686.
For more information, please contact:
David Rupp, Oregon State University